To ‘green’ AI, scientists are making it less resource-hungry
Curbing AI’s use of energy and water could seriously lessen its threat to our climate
By Kathryn HulickComputing equipment stacked all the way to the ceiling. Thousands of little fans whirring. Colored lights flashing. Sweltering hot aisles alongside cooler lanes. Welcome to a modern data center.
Every ChatGPT conversation, every Google search, every TikTok video makes its way to you through a place like this.
“You have to go in with a jacket and shorts,” says Vijay Gadepally with the Massachusetts Institute of Technology, or MIT. As a computer scientist at MIT’s Lincoln Laboratory in Lexington, Mass., he helps run a data center that’s located a couple of hours away by car in Holyoke. It focuses on supercomputing. This technology uses many powerful computers to perform complex calculations.
Entering the data center, you walk past a power room where transformers distribute electricity to the supercomputers. You hear “a humming,” Gadepally says. It’s the sound of the data center chowing down on energy.
Data centers like this are very hungry for electricity and their appetites are growing. Most are also very thirsty. Cooling their hardworking computers often takes loads of fresh water.
More people than ever before are using applications that rely on supercomputers, says Gadepally. On top of that, he adds, supercomputers are doing more energy-intensive things. Stuff like running ChatGPT. It’s an artificial intelligence, or AI, model that can generate code, write text or answer questions. Some scientists estimate that answering a question with ChatGPT or a similar AI tool consumes about 10 times as much electricity as a Google search.
Just two months after it launched, ChatGPT reached 100 million active users, making it the fastest growing app ever. And, Gadepally adds, energy-hungry AI doesn’t just power chatbots. “AI is making its way into everything.” Generating one image using an AI model such as Stable Diffusion can draw as much energy as fully charging a smartphone. That’s the recent finding of researchers at a collaborative AI platform called Hugging Face.
Meanwhile, the climate crisis is worsening. Since people still burn fossil fuels to produce most of our electricity, a growing demand for energy leads to higher releases of greenhouse gases. That’s got some experts looking at how to cut the climate impact of AI. Their goal: to make such increasingly popular AI tools more sustainable.
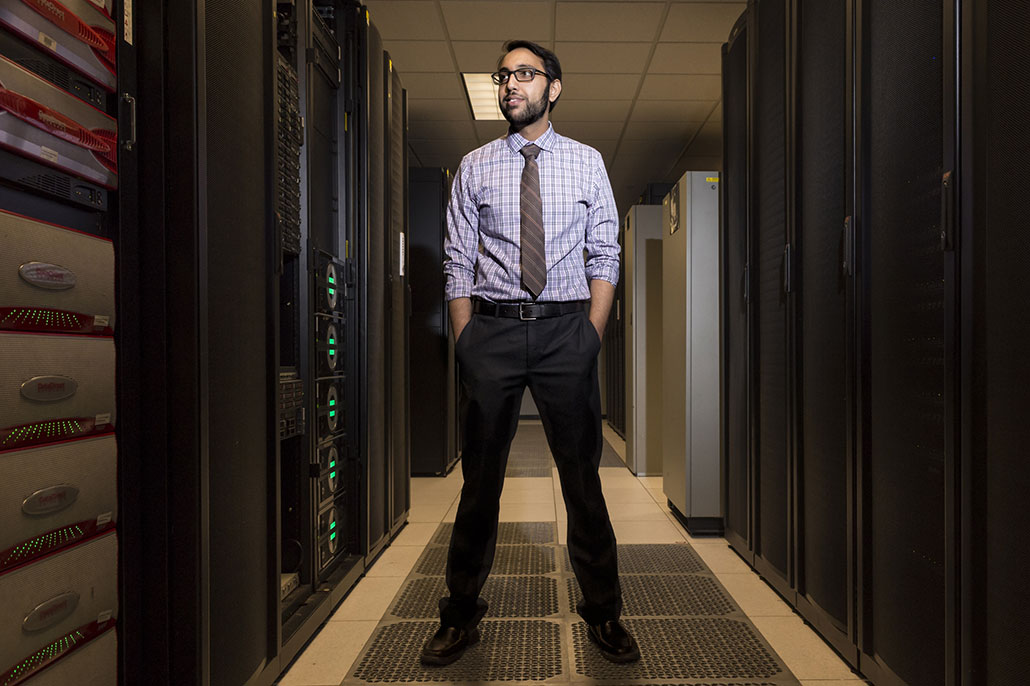
Vijay Gadepally helps manage a group of supercomputers located at the Lincoln Laboratory Supercomputing Center in Holyoke. “A lot of the Massachusetts universities utilize this as their data center,” he says. His team has found ways to make their supercomputers devour less energyMIT LINCOLN LABORATORY
AI’s appetite for energy depends on what type of model it is. Many of the ones used in scientific research are quite small. “A lot of the models I’ve trained take a few hours on a personal computer,” says Alex Hernandez-Garcia. This AI expert works as a researcher at Mila, an AI institute in Montreal, Canada. A lean model like that has a teeny-tiny carbon footprint, he says. It may be similar to the power used to keep an incandescent light bulb lit for a few hours.
However, tools like ChatGPT rely on large language models, or LLMs. An LLM is a type of AI based on machine learning. It learns to predict the order of words.
As their name implies, LLMs are big. Really big. Because there is so much language data available to feed them, they tend to be the largest of all machine-learning models. It takes months and many supercomputers to train them, says Hernandez-Garcia.
In a 2023 paper, his team surveyed the carbon footprints of many AI models. Based on this research, he estimated the climate impact of training the LLM GPT-3. (Updated versions of this model run ChatGPT today). Its impact might equal some 450 commercial airplane flights between London and New York City, he found. This research also looked at models trained to classify images, detect objects, translate languages and more.
Making any of these models bigger often provides better results. But a large jump in model size usually leads to only a very tiny increase in its ability, notes Hernandez-Garcia. Bigger isn’t always better, his team has shown. Models whose use led to the most greenhouse-gas emissions didn’t always perform the best, their analysis showed.
In a 2021 paper, Emily M. Bender argued that, in fact, LLMs may be getting too big. Bender is a computational linguist at the University of Washington in Seattle. “AI is a luxury,” she says. Therefore, people should think carefully about the ethics of building ever-larger models.
Bigger isn’t always better
AI’s appetite for energy depends on what type of model it is. Many of the ones used in scientific research are quite small. “A lot of the models I’ve trained take a few hours on a personal computer,” says Alex Hernandez-Garcia. This AI expert works as a researcher at Mila, an AI institute in Montreal, Canada. A lean model like that has a teeny-tiny carbon footprint, he says. It may be similar to the power used to keep an incandescent light bulb lit for a few hours.
However, tools like ChatGPT rely on large language models, or LLMs. An LLM is a type of AI based on machine learning. It learns to predict the order of words.
As their name implies, LLMs are big. Really big. Because there is so much language data available to feed them, they tend to be the largest of all machine-learning models. It takes months and many supercomputers to train them, says Hernandez-Garcia.
In a 2023 paper, his team surveyed the carbon footprints of many AI models. Based on this research, he estimated the climate impact of training the LLM GPT-3. (Updated versions of this model run ChatGPT today). Its impact might equal some 450 commercial airplane flights between London and New York City, he found. This research also looked at models trained to classify images, detect objects, translate languages and more.
Making any of these models bigger often provides better results. But a large jump in model size usually leads to only a very tiny increase in its ability, notes Hernandez-Garcia. Bigger isn’t always better, his team has shown. Models whose use led to the most greenhouse-gas emissions didn’t always perform the best, their analysis showed.
In a 2021 paper, Emily M. Bender argued that, in fact, LLMs may be getting too big. Bender is a computational linguist at the University of Washington in Seattle. “AI is a luxury,” she says. Therefore, people should think carefully about the ethics of building ever-larger models.
The worst-case scenario
One measure of an AI model’s size is the number of parameters it contains. Parameters are what get tweaked as the model learns. The more parameters a model has, the more detail it can learn from data. That often leads to higher accuracy.
GPT-2 — an LLM from 2019 — had 1.5 billion parameters. Just a couple years later, GPT-3.5 was using 175 billion parameters. The free version of ChatGPT runs on that model today. Users who pay for the app now get access to GPT-4, an even more advanced LLM. It’s said to manipulate an estimated 1.7 trillion parameters!
The free version of ChatGPT that was running in early 2023 was the one that consumed about 10 times as much energy per question as Google, says Alex de Vries. He’s a PhD student in economics at Vrije (Public) University Amsterdam in the Netherlands. He’s also the founder of Digiconomist. This company studies the impact of digital trends.
In a 2023 study, de Vries estimated that at the height of ChatGPT’s popularity, the app was likely consuming about 564 megawatt hours of electricity per day. That’s roughly equal to the daily energy use of about 19,000 U.S. households. So he decided to do a thought experiment: What if every Google search people are doing right now instead went through an LLM such as ChatGPT? “Google alone would be consuming as much power as Ireland,” he realized.
Will AI tools based on giant, energy-hungry LLMs soon gobble up as much electricity as entire countries? Not overnight.
The good news, de Vries says, is that his thought experiment is “an extreme example.” Most tech companies, he notes, can’t afford to buy that much energy. Plus, data centers don’t have enough supercomputers to support such a huge demand for AI. This type of AI requires special computer chips. Right now, factories can’t make those chips fast enough, he says. “That gives us some time to reflect on what we’re doing” — and maybe do things differently.
![]() |
As this video notes, the electricity-hungry computers that make AI possible could put enough demand on fossil fuels to pose a big threat via global warming — and possibly cause some governments to take action. Or at least that’s one take-home lesson from a similar threat posed by cryptocurrency mining. |
Putting data centers on a diet
Gadepally and his team aren’t just reflecting — they’re acting. They’ve found several ways to put their data center on an energy diet.
Not all AI tasks require a humongous energy hog, the Hugging Face study showed. These researchers measured the carbon footprint of small models trained only to perform a single task, such as tagging movie reviews as either positive or negative. The footprint of tagging 1,000 reviews with a small model was around 0.3 gram of carbon dioxide, or CO2. When the researchers did the same task with big, powerful LLMs, they found emissions of around 10 grams of CO2 — 30 times as much.
Gadepally’s team has developed a new AI model that could help rein in other AI models. Called CLOVER, it figures out what a user is trying to do, then selects only as big a model as that task truly needs.
CLOVER can “mix and match models to best suit the task at hand,” says Gadepally. This year, his team reported that CLOVER can cut the greenhouse-gas emissions of AI use at a data center by more than 75 percent. With those savings, the accuracy of the results that AI models provide drops by only 2 to 4 percent.
Video games provided the idea for another energy-saving trick. “One of our colleagues is a big gamer,” notes Gadepally. Machine-learning models run on what’s known as graphics processing units, or GPUs. High-end video games use this same type of computer chip. His colleague found he could put a brake on the power his GPU could draw while playing games. Scientists refer to this tactic as “power capping.” Usually, it does not impact the quality of games running on GPUs.
As GPUs work harder, they draw more power — and heat up. If they aren’t allowed to draw as much power at once, their work may take a bit longer. But power-capped GPUs aren’t wasting energy ramping up and then slowing back down, the way non-capped GPUs do. Plus, power-capped GPUs don’t get as hot. That means they also don’t need as much cooling.
Gadepally’s team tested this with an LLM named BERT. Without power-capping, it took 79 hours to train BERT. With power-capping, training took three hours more. But they saved energy, he says, equal about to what’s used in a week by the average U.S. household. That’s a big energy savings for a small amount of added time.
Their tests were so successful that they’re now using power-capping throughout the data center. “Some people have said we’re a bit weird for doing it,” says Gadepally. But he hopes others will follow their lead.
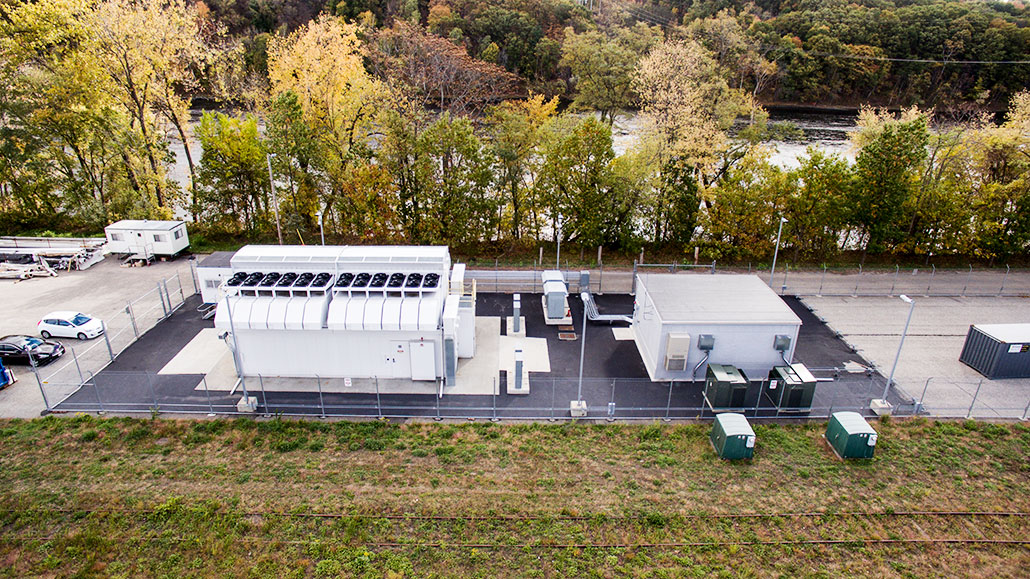
How to ‘imagine AI differently’
The data center where Gadepally’s group did all these tests actually has a fairly small carbon footprint. That’s because its electricity mainly comes from a nearby hydroelectric dam. This is a water-powered energy source that doesn’t release much greenhouse gas into the air. Tech companies can reduce their climate impact by building data centers or scheduling data calculations at places that get most of their power from renewable sources.
However, there’s only so much green energy to go around. Using it for AI means not using it for something else.
Also, the best places to collect green energy may not be ideal for data centers. Arizona is a state where a lot of solar and wind farms already feed electricity into the power grid. The state’s weather, however, is very hot. Data centers everywhere need to keep their computers from overheating. Most use fresh water to do this.
“Computing needs a tremendous amount of water,” points out Shaolei Ren. He’s a computer engineer at the University of California, Riverside. Climate change is making fresh water scarcer, especially in places like Arizona. So thirsty data centers built in those areas can become a big problem.
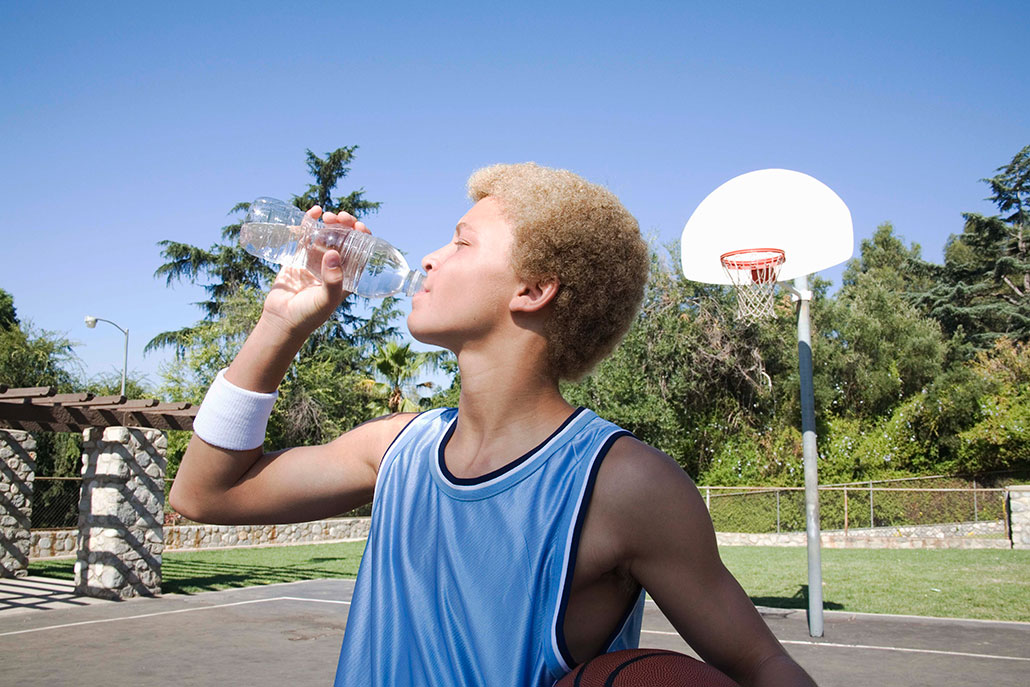
Hernandez-Garcia, Ren and other experts have called for tech companies to measure and report on their greenhouse-gas emissions and water footprints. That’s a great idea. But there’s only so much that tech companies can do to cut these impacts while building ever-larger AI models.
Real change starts deeper, with the way society approaches the systems it builds, suggests Priya Donti. Before throwing all available resources into a system, we need to consider that system’s sustainability as well as its environmental and social impact. Donti is a computer scientist at MIT in Cambridge, Mass. She also co-founded the organization Climate Change AI. This group looks at ways AI and machine learning can help society reach climate goals.
Right now, says Donti, large tech companies are driving the emergence of ever bigger AI models. But “it doesn’t have to be that way,” she says.
Researchers are finding creative ways to make smart, useful, greener AI. For example, they can transfer insights between AI models. They also can train using less — but higher quality — data.
One company, Numenta, is looking to the human brain for inspiration. Designing AI models that are more similar to the brain means “much less math has to be done,” explains co-founder Jeff Hawkins. And fewer calculations means a lower demand for energy.
“AI doesn’t have to be super, super data-hungry or super, super compute-hungry,” says Donti. Instead, we can “imagine AI differently.”
Power Words:
app: Short for application, or a computer program designed for a specific task.
artificial intelligence: A type of knowledge-based decision-making exhibited by machines or computers. The term also refers to the field of study in which scientists try to create machines or computer software capable of intelligent behavior.
average: (in science) A term for the arithmetic mean, which is the sum of a group of numbers that is then divided by the size of the group.
carbon footprint: A popular term for measuring the global warming potential of various products or processes. Their carbon footprint translates to the amount of some greenhouse gas — usually carbon dioxide — that something releases per unit of time or per quantity of product.
climate: The weather conditions that typically exist in one area, in general, or over a long period.
climate change: Long-term, significant change in the climate of Earth. It can happen naturally or in response to human activities, including the burning of fossil fuels and clearing of forests.
code: (in computing) To use special language to write or revise a program that makes a computer do something. (n.) Code also refers to each of the particular parts of that programming that instructs a computer's operations.
colleague: Someone who works with another; a co-worker or team member.
commercial: An adjective for something that is ready for sale or already being sold. Commercial goods are those caught or produced for others, and not solely for personal consumption.
computational: Adjective referring to some process that relies on a computer’s analyses.
computer chip: (also integrated circuit) The computer component that processes and stores information.
data: Facts and/or statistics collected together for analysis but not necessarily organized in a way that gives them meaning. For digital information (the type stored by computers), those data typically are numbers stored in a binary code, portrayed as strings of zeros and ones.
data center: A facility that holds computing hardware, such as servers, routers, switches and firewalls. It also will house equipment to support that hardware, including air conditioning and backup power supplies. Such a center ranges in size from part of a room to one or more dedicated buildings. These centers can house what it takes to make a “cloud” that makes possible cloud computing.
digital: (in computer science and engineering) An adjective indicating that something has been developed numerically on a computer or on some other electronic device, based on a binary system (where all numbers are displayed using a series of only zeros and ones).
economics: The social science that deals with the production, distribution and consumption of goods and services and with the theory and management of economies or economic systems. A person who studies economics is an economist.
electricity: A flow of charge, usually from the movement of negatively charged particles, called electrons.
engineer: A person who uses science and math to solve problems. As a verb, to engineer means to design a device, material or process that will solve some problem or unmet need.
focus: (in behavior) To look or concentrate intently on some particular point or thing.
fossil fuel: Any fuel — such as coal, petroleum (crude oil) or natural gas — that has developed within the Earth over millions of years from the decayed remains of bacteria, plants or animals.
graphics processing unit (GPU): A type of computer processor that can be programmed to depict the graphics needed for a realistic video game.
green: (in chemistry and environmental science) An adjective to describe products and processes that will pose little or no harm to living things or the environment.
grid: (in electricity) The interconnected system of electricity lines that transport electrical power over long distances. In North America, this grid connects electrical generating stations and local communities throughout most of the continent.
insight: The ability to gain an accurate and deep understanding of a situation just by thinking about it, instead of working out a solution through experimentation.
large language models: Computer programs that use machine learning to interpret human languages so that they can predict or generate statements. To do this, such systems must first analyze — evaluate and learn from — enormous data sets (such as books or even whole libraries).
machine learning: A technique in computer science that allows computers to learn from examples or experience. Machine learning is the basis of some forms of artificial intelligence (AI). For instance, a machine-learning system might compare X-rays of lung tissue in people with cancer and then compare these to whether and how long a patient survived after being given a particular treatment. In the future, that AI system might be able to look at a new patient’s lung scans and predict how well they will respond to a treatment.
model: A simulation of a real-world event (usually using a computer) that has been developed to predict one or more likely outcomes. Or an individual that is meant to display how something would work in or look on others.
parameter: A conditions of some situation to be studied or defined that can be quantified or in some way measured.
PhD: (also known as a doctorate) A type of advanced degree offered by universities — typically after five or six years of study — for work that creates new knowledge. People qualify to begin this type of graduate study only after having first completed a college degree (a program that typically takes four years of study).
renewable energy: Energy from a source that is not depleted by use, such as hydropower (water), wind power or solar power.
scenario: A possible (or likely) sequence of events and how they might play out.
society: An integrated group of people or animals that generally cooperate and support one another for the greater good of them all.
solar: Having to do with the sun or the radiation it emits. It comes from sol, Latin for sun.
survey: To view, examine, measure or evaluate something, often land or broad aspects of a landscape.
sustainable: (n. sustainability) An adjective to describe the use of resources in a such a way that they will continue to be available long into the future.
system: A network of parts that together work to achieve some function. For instance, the blood, vessels and heart are primary components of the human body's circulatory system. Similarly, trains, platforms, tracks, roadway signals and overpasses are among the potential components of a nation's railway system. System can even be applied to the processes or ideas that are part of some method or ordered set of procedures for getting a task done.
tactic: An action or plan of action to accomplish a particular feat.
technology: The application of scientific knowledge for practical purposes, especially in industry — or the devices, processes and systems that result from those efforts.
transformer: (in physics and electronics) A device that changes the voltage of an electrical current.
trillion: A number representing a million million — or 1,000,000,000,000 — of something.
unit: (in measurements) A unit of measurement is a standard way of expressing a physical quantity. Units of measure provide context for what numerical values represent and so convey the magnitude of physical properties. Examples include inches, kilograms, ohms, gauss, decibels, kelvins and nanoseconds.
weather: Conditions in the atmosphere at a localized place and a particular time. It is usually described in terms of particular features, such as air pressure, humidity, moisture, any precipitation (rain, snow or ice), temperature and wind speed. Weather constitutes the actual conditions that occur at any time and place. It’s different from climate, which is a description of the conditions that tend to occur in some general region during a particular month or season.
CITATIONS
Journal: A.S. Luccioni, Y. Jernite and E. Strubell. Power hungry processing: Watts driving the cost of AI deployment? arXiv. November 28, 2023, 20 pp. doi: 10.48550/arXiv.2311.16863.
Journal: P. Li et al. Making AI less "thirsty": Uncovering and addressing the secret water footprint of AI models. Arxiv. October 29, 2023. doi: 10.48550/arXiv.2304.03271.
Journal: A. de Vries. The growing energy footprint of artificial intelligence. Joule. Vol. 7, October 18, 2023, p. 2191. doi: 10.1016/j.joule.2023.09.004.
Journal: B. Li et al. Clover: Toward sustainable AI with carbon-aware machine learning inference service. Arxiv. August 31, 2023. doi: 10.48550/arXiv.2304.09781.
Journal: A.S. Luccioni and A. Hernandez-Garcia. Counting carbon: A survey of factors influencing the emissions of machine learning. Arxiv. February 16, 2023. doi: 10.48550/arXiv.2302.08476.
Journal: A.S. Luccioni, S. Viguier and A.-L. Ligozat. Estimating the carbon footprint of BLOOM, a 176B parameter language model. Arxiv. November 3, 2022. doi: 10.48550/arXiv.2211.02001.
Journal: J. McDonald et al. Great power, Great responsibility: Recommendations for reducing energy for training language models. Arxiv. May 19, 2022. doi: 10.48550/arXiv.2205.09646.
Journal: D. Patterson et al. The carbon footprint of machine learning training will plateau, then shrink. Arxiv. April 11, 2022. doi: 10.48550/arXiv.2204.05149.
About Kathryn Hulick
Kathryn Hulick is a freelance science writer and the author of Strange But True: 10 of the World's Greatest Mysteries Explained, a book about the science of ghosts, aliens and more. She loves hiking, gardening and robots.
Source: [ ScienceNewsExplores ]
Note: [Content may be edited for style and length.]